Nonparametric Statistics: Overview & Examples
The field of statistics can be incredibly complicated.
There are many different tried and tested methods that can be used to analyze data gathered from different areas.
Nonparametric statistics is a great tool to use when certain factors come into play. Whether the sample size is too small or the analyzed data is ordinal or nominal. Or even the underlying data does not meet the assumptions.
Read on as we go into more depth and detail on nonparametric statistics.
Table of Contents
KEY TAKEAWAYS
- Nonparametric statistics is a method of statistics. This is in which data is not assumed to come from prescribed models.
- They are an easy to use method of statistics but don’t offer the same pinpoint accuracy that other statistical methods have.
- This type of analysis is commonly suited to when you’re considering the order of something. Specifically, even if the number data changes, the results are likely to stay the same.
What Is Nonparametric Statistics?
Nonparametric statistics is a method of statistics that makes statistical inferences. This is without regard to any distribution that is underlying. The data used is not assumed to come from any prescribed models. These are models that are determined from a small sample of parameters. For example the normal distribution model and the linear regression model.
The data that is often used in nonparametric statistics would be data that is ordinal. This means that it doesn’t necessarily rely on numbers, but rather on an order or a ranking.
For example, a survey conducted to ask people to rate something from ‘Like’ to ‘Dislike’. This is ordinal data.
Nonparametric statistics also include nonparametric descriptive statistics and statistical models. As well as inference and statistical tests.
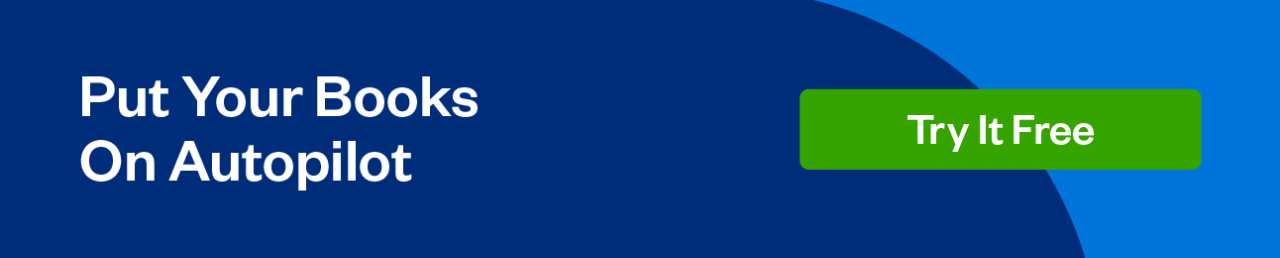
What Does Nonparametric Mean?
Simply put, the term nonparametric doesn’t mean that the model structure lacks parameters. In fact, it means that the nature and the number of the parameters are flexible, therefore are not fixed in advance.
How Do Nonparametric Statistics Work?
Parametric statistics include parameters. These parameters could include the mean or the Pearson correlation. Or it could be variance or standard deviation, etc.
This form of statistics works by using observed data. This in order to estimate the parameters of the distribution.
For parametric statistics, data is often assumed to have come from a standard distribution. This is a distribution that has unknown parameters. This is normally written as μ, which is the population mean, and σ2 which is the population variance.
However, nonparametric statistics doesn’t make assumptions. Specifically, assumptions that any data is taken from a normal distribution. The shape of the distribution is instead estimated under this form of statistical measurement. There are many situations where a normal distribution can be assumed. But there are also a number of different scenarios where the true data generating process is in no way normally distributed.
The Types of Nonparametric Statistics
There are two different types of nonparametric statistical methods.
The first type aims to discover the unknown underlying distribution of data. This is the data that has been observed. The second method aims to make a statistical inference regarding the underlying distribution.
For the first approach, histograms and kernel methods are commonly used to try and figure out the values of the parameters. Histograms involve testing hypotheses without the literal data values. Instead, it is based on the rank ordering of the data in question.
Nonparametric statistics are commonly easier to apply when compared to parametric statistics. This is due to the lack of assumption involved.
No assumptions are made during a standard mathematical procedure for hypothesis testing. This would be about the probability distributions. Which would include distribution sign tests, t-tests, and single-population inferences.
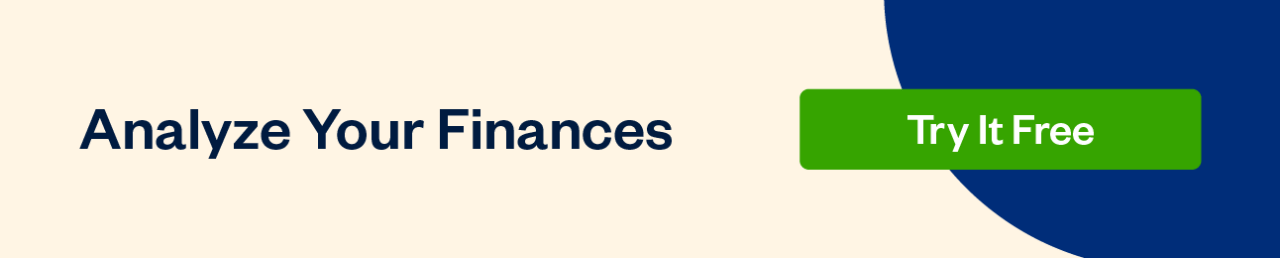
What Are Some Examples of Nonparametric Statistics?
Let’s say that a financial analyst wants to estimate the value-at-risk (VaR) of one of their potential investments. The analyst would gather earnings data from a large sample set of similar investments that have occurred over a similar period of time.
Rather than assuming that the earnings follow a normal distribution, the analyst would use the histogram. This would be to try and figure out the distribution nonparametrically. This histogram would then be able to provide the analyst with a nonparametric estimate of the value-at-risk.
For a second example, let’s say that a researcher wants to be able to estimate the number of babies that will be born left-handed in the state of Ohio.
The researcher would perform an analysis of the data by taking a sample of 500 babies born within the state. They would note how many were born as left-handed dominant. They would then take an estimate of the entire population of babies born left-handed the following year. This would be the derived measurement.
The sample size is assumed to be chosen randomly. And the number of left-handed babies can be assumed to be normal. However, the experiment that measures the number of babies to be born a certain way cannot be assumed to take on a normal distribution.
What Is the Difference Between Parametric and NonParametric Statistics?
The main difference between parametric and nonparametric statistics is based on what assumptions are made. Parametric statistics is based on assumptions about the distribution of the population. This is where the sample was taken.
However, nonparametric statistics are not actually based on assumptions at all. Meaning that the data can be taken from a sample that doesn’t follow a specific distribution.
Summary
The nonparametric statistics approach has a number of weaknesses and strengths. But when compared to parametric tests, nonparametric tests can be more potent. However, nonparametric statistics are a substitute method. This is due to the low degree of confidence that would have been obtained from earlier statistical results.
Nonparametric statistics are commonly used because of the ease at which they can be applied. It can also be used when there is an absence of vital information such as the sample size, standard deviation, or the mean. This gives it a broader scope of application.
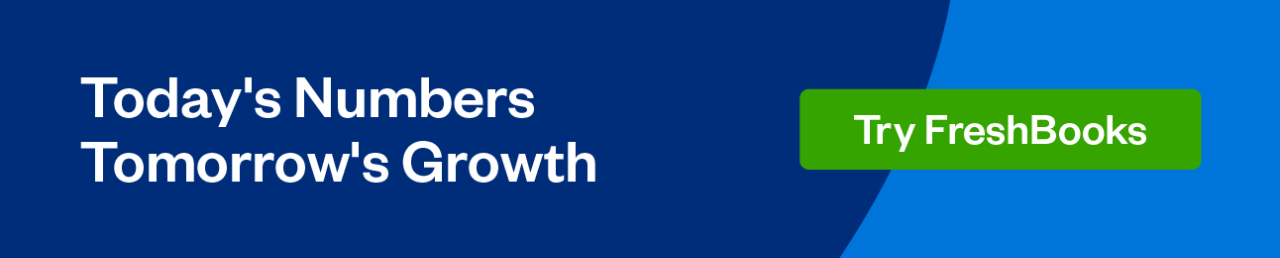
FAQs on Nonparametric Statistics
A nonparametric method refers to a method where the data isn’t assumed to have come from a prescribed model. These are models that can be determined by parameters of a smaller number.
One of the disadvantages of this method is that it is less efficient when compared to parametric testing. The results gathered by nonparametric testing may or may not provide accurate answers. This is because they are distribution free.
The advantages of this method are that they make fewer assumptions about the data. They are also useful for analyzing data that is set in ranks or categories and not necessarily by numbers.
Common assumptions of a nonparametric test are independence and randomness.
Share: