Heteroskedasticity: Definition, Overview & Example
Understanding data sets will provide you with a broad range of valuable information. You can get a deeper sense as to how certain things are working together, and also if there are any variations.
But what happens when you have a broad variance of values that aren’t consistent? Well, this is when heteroskedasticity occurs. Are you sitting there and wondering how this works and why it’s important? Good! We created this guide just for you. Read on to learn more!
Table of Contents
KEY TAKEAWAYS
- Heteroskedasticity consistently occurs when there’s a variance not constant across all values.
- Heteroskedasticity can create problems when estimating regression models.
- There are several ways to test for heteroskedasticity, including the Breusch-Pagan test and the White test.
What Is Heteroskedasticity?
Heteroskedasticity is a measure of variation of the residual standard deviation in regression models. It can be seen as a measure of how much the variance of the error term (residuals) in a linear regression model deviates from sampling variance and therefore cannot be explained solely by sampling variation.
Moreover, heteroskedasticity presents a problem when performing regression analysis, because it indicates that the variance of our response variable is not constant across all coefficients (independent variables).
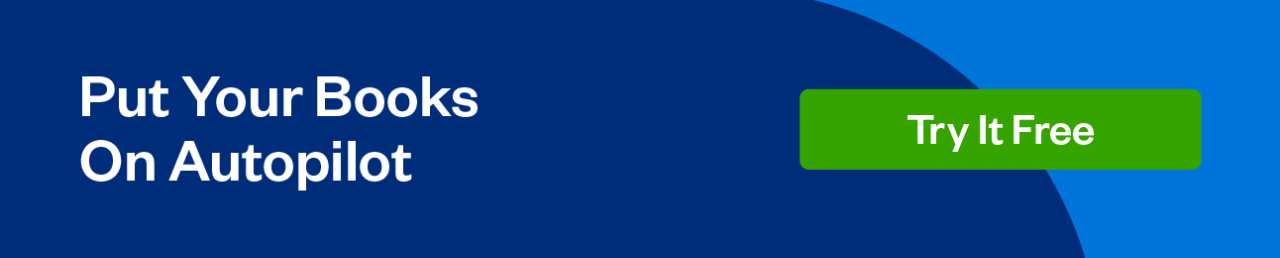
Types of Heteroskedasticity
There are two main types of heteroskedasticity:
Unconditional Heteroskedasticity
This occurs when the variance of the dependent variable is not constant across all values of the independent variable.
Conditional Heteroskedasticity
This occurs when the variance of the dependent variable is not constant across all values of the predictor variables. But after taking into account the other predictor variables in the model.
Unconditional heteroskedasticity is more common than conditional heteroskedasticity. But both can create problems when estimating regression models.
Causes of Heteroskedasticity
There are several potential causes of heteroskedasticity, including:
Omitted variables: If there are important variables that are not included in the model, this can lead to heteroskedasticity.
Misspecified functional forms: If the functional form of the model is not correctly specified, this can also lead to heteroskedasticity.
Incorrect model specification: If the model’s misspecified in other ways. Such as by using the wrong type of regression or by failing to include an interaction term. This can also lead to impure heteroskedasticity.
Random error: In some cases, heteroskedasticity may be due to a random variable error and cannot be corrected.
There are several methods that can correct for heteroskedasticity, including:
Winsorization: This involves replacing all values that are above or below a certain threshold with the threshold value.
Weighted least squares regression: This approach weights the observations by their variances. This helps to reduce the impact of heteroskedasticity on the estimates.
Huber estimator: This is a robust estimation technique that is less affected. Outliers and heteroskedasticity work instead of traditional methods.
Use robust standard errors: Robust standard errors are less affected than a traditional standard error term.
Transformed dependent variable: In some cases, transforming the dependent variable can help to reduce the impact of heteroskedasticity.
Use a different estimator: There are some estimators, such as the generalized least squares estimator. They are less affected by heteroskedasticity than traditional methods.
The presence of heteroskedasticity can have a significant impact on regression models. This causes the standard errors of the estimates to be incorrect. This can lead to incorrect conclusions about the significance of the estimates and about the hypotheses test results. Heteroskedasticity can also cause problems with predictions made using the regression equation.
The chances of heteroskedasticity are a potential problem when estimating regression models. But it can be corrected using a variety of methods. It is important to be aware of the impact of heteroskedasticity on regression models and to test for it when estimating a model.
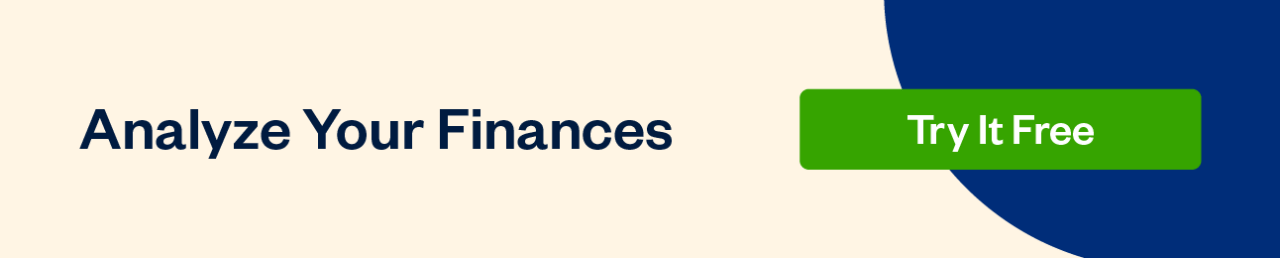
Why is Homoscedasticity Important?
Having a regression model that is homoskedastic is important because it allows us to make consistent forecasts and valid conclusions. If your model is non-linear, you cannot trust the standard model diagnostics and you cannot make predictions outside of the range of data points used for fitting the model.
If your model is heteroskedastic, you cannot trust the standard error estimates and you cannot make consistent forecasts outside of the range of data points used for fitting the model.
If the model is heteroskedastic, then we are likely to over- or under-estimate the impact of the coefficients. Having a regression model that is homoskedastic allows us to make reliable forecasts and valid conclusions about our data.
Summary
Heteroskedasticity is a problem that can occur with regression analysis. It is a measure of the degree to which the variance of the error term in a linear regression model is not constant across all coefficients (independent variables).
This means that the relationship between the independent variables and the response variable is non-linear. The presence of heteroskedasticity in your model means that you cannot trust any standard model diagnostics (such as the F-test and R-squared).
You cannot draw valid conclusions or make reliable predictions from your model. Having a regression model that is homoskedastic is important because it allows us to make consistent forecasts and valid conclusions.
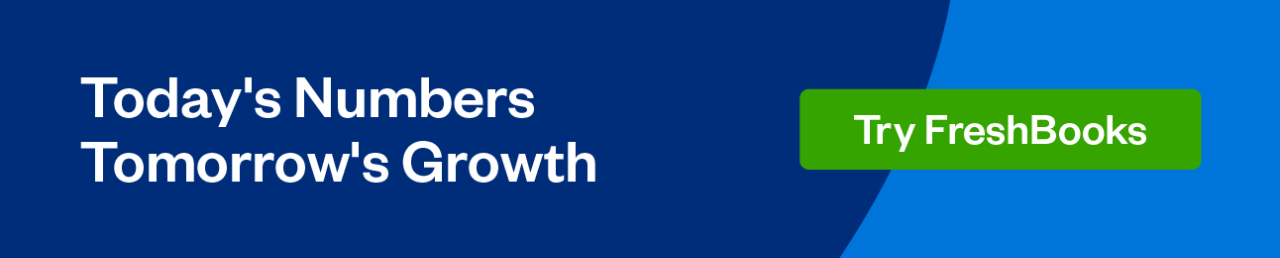
FAQs About Heteroskedasticity
Heteroskedasticity is not necessarily good or bad. But it can cause problems with the standard errors of the estimates if it is not taken into account.
Heteroskedasticity is when the variance of the residuals is not constant. Homoskedasticity is when the variance of the residuals is constant.
There is no one “best” test for heteroskedasticity. But some common tests include the Breusch-Pagan test, the White test, and the Goldfeld-Quandt test.
Some methods for correcting for heteroskedasticity include transforming the dependent variable.
Ways to deal with heteroskedasticity in regression include transforming the dependent variable.
Share: